Introduction
Breast cancer has become the most common cancer in women in the latest data of global cancer [1]. The 5-year survival rate of breast cancer patients varied significantly with stage of initial diagnosis (98% for stage I and 27% for stage IV) [2]. Thus, early detection of breast cancer is crucial [3]. Ultrasound (US) has been one of the most widely used modalities for breast cancer diagnosis owing to it being noninvasive and nonradiative, convenient, and cost-effective [4]. In addition, to narrow variability in characterization and final assessment of breast lesions identified on ultrasonography, the Breast Imaging Report and Data System (BI-RADS®) proposed by the American College of Radiology (ACR) unified and standardized the US lexicon and classification [5].
US could assess the morphologies, orientations, internal structures, and margins of lesions from multiple planes with high resolution both in predominantly fatty breasts and in dense glandular structures [6]. However, sonographic manifestations of the lesions varied in different sections due to different growth patterns of the lesions and the influences of surrounding tissue compositions [7]. For example, ductal carcinoma in situ (DCIS) was reported to grow faster in the radial direction than in the anti-radial plane [8]. The visualizations of handheld US images commonly used as documentation usually depended on the information collected in one certain orientation, which was empirically selected by the operator [9]. Thus, the features of lesions reflected only in the static images were likely to be inaccurate. Besides, the examiner could gain important information about surrounding tissue characteristics of the lesions by dynamic US scanning. This information, however, was likely incomplete when the lesion was documented as static images.
In theory, these shortcomings of static image documentation could be overcome by recording the breast lesion as videos [10]. To date, there had been only two studies comparing video and static images of breast lesions with respect to sonographic assessment and diagnostic performance. An article reported that the echogenic halo, orientation, and margin varied while comparing video and static images [11]. Meanwhile, another study showed that the inter-observer variability and diagnostic performance of video clips were similar to those of static images [12]. Thus, whether dynamic observation is superior to static observation needs further study.
Regarding the possible discrepancy ultrasonographic features in different sections of the lesions, we hypothesized that the benign and malignant tendencies of the lesions probably were not consistent when lesions were viewed in different directions. Previous studies found that the results of the quantitative evaluation of breast magnetic resonance imaging and elastography in different directions were different for some atypical lesions, especially malignant lesions. The role of section discrepancy was also explored in the identification of benign and malignant lesions using elastography [13–16]. Nevertheless, whether this rule was applicable in 2D US remains to be studied.
To the best of our knowledge, there was no research comparing diagnostic performance and consistency of dynamic videos and static images between different sections. Therefore, the purposes of this study were to investigate if there were section discrepancy in 2D US and to investigate the diagnostic performance of 2D US based on dynamic videos and static images of breast lesions.
Materials and methods
Patients
The institutional review board approved this retrospective study, and the requirement for informed consent was waived. From September 2018 to April 2020, 723 consecutive women scheduled for breast surgery underwent breast US examinations. The inclusion criteria were presence of a solid mass that occupied space and could be detected in two perpendicular planes with US and available final pathology. The exclusion criteria are presented in Figure 1 . Finally, 449 women with 468 breast lesions were included in the study.
Image acquisition
All examinations were performed using the US device Oxana2 (ACUSON Oxana Series; Siemens, Munich, Germany). The frequency range of the probe was 6-12 MHz. All the examinations were performed by the same doctor, who have more than 15 years of experience in breast US. For each lesion, at least three static images were obtained: two representative two-dimensional (2D) static images in two perpendicular planes (horizontal and sagittal) and an image with marked maximum diameter and depth of the lesion. Two corresponding real-time scans of the breast lesion based on horizontal and sagittal planes were collected. The scan range of each video was from “normal glandular tissue–lesion emergence–lesion complete display–lesion disappearance–normal glandular tissue.” Each dynamic videos lasted for about 10 seconds. All the static images and dynamic videos were saved for further analysis.
Image evaluation
Independent and blinded image evaluation were performed by two observers with 15 and 3 years of breast sonography experience, respectively. All the data were verified, and any conflict was resolved by consensus. For lesion assessment, the Breast Imaging and Reporting Data System (BI-RADS®) US lexicon [5] was used. Suspicious US features were defined based on previous studies and the criteria for BI-RADS 4 subcategorization were established according to Li’s research [17]. Suspicious 2D characteristics were divided into malignant and auxiliary malignant signs. Malignant signs included irregular shape, nonparallel orientation, non-circumscribed margin (indistinct, spiculated, microlobulated, or angular), posterior shadowing, microcalcification in the mass, and intraductal calcification. Auxiliary malignant signs included round shape, hypoechoic, abundant blood flow signal, and architectural distortion [18–21]. Category 5 was defined as lesions with at least three malignant signs or two malignant signs plus two or more auxiliary malignant signs. Category 4C was defined as lesions with two malignant signs with or without one auxiliary malignant sign. Category 4B was defined as lesions with one malignant sign and two or more auxiliary malignant signs. Category 4A was defined as lesions with one malignant sign plus one auxiliary malignant sign or with two or more auxiliary malignant signs without any malignant sign. A hypoechoic lesion without any suspicious signs was defined as category 3. Category 3 was used as the cut-off point in this study according to the different clinical management because tissue diagnosis was recommended for category ≥4 by ACR BI-RADS. Therefore, lesions with categories 3 were considered benign; lesions with categories 4A, 4B, 4C, or 5 were considered malignant in the study. If both sections of a lesion showed the same character (benign or malignant), no section difference was defined. If the two planes of a lesion showed different character, section difference was defined.
Statistical analysis
Receiver operating characteristics (ROC) curves were constructed, and the areas under the ROC curves (AUCs) were compared to evaluate the diagnostic efficacies of 2D static images and dynamic videos [22]. Sensitivity, specificity, accuracy, positive predictive value, and negative predictive value were calculated separately using category 3 as the cut-off point. Chi-square test was used to check whether these indicators were different. To evaluate the variations of different sections in lesion description (US features) and assessment (BI-RADS® categories), Pearson’s chi-square test or Fisher’s exact test, where appropriate, was used to analyze these categorical data. The contingency coefficient was used for correlation analysis of disordered classification variable. Chi-square test was used to analyze the difference of categorical variables, whereas t test was used to analyze the difference of continuous variables. P < 0.05 was considered statistically significant. All data were analyzed with the SPSS version 20.0 (IBM Corp., Armonk, NY, USA) and MedCalc version 19.3 (MedCalc, Mariakerke, Belgium).
Results
Patients and lesions characteristics
In total, 449 patients (mean age, 42.8 ± 12.7 years; range, 14–88 years) with 468 breast lesions were included. Of 449 patients, 200 (42.7%) were younger than 40 years, whereas 249 (57.3%) were 40 years or older. There were 315 benign lesions and 153 malignant lesions. The lesion sizes ranged from 3.7 to 38.0 mm (mean, 13.7 ± 6.2 mm). The pathological results of the lesions are summarized in Table 1 .
Pathology of the Lesions
Benign | 315 (67.3) | Malignant | 153 (32.6) |
Fibroadenoma | 168 (53.3) | Invasive ductal carcinoma | 107 (69.9) |
Fibrocystic disease | 92 (29.2) | Ductal carcinoma in situ | 13 (8.4) |
Intraductal papilloma | 29 (9.2) | Papillary carcinoma | 9 (5.8) |
Benign phyllodes tumor | 7 (2.2) | Invasive lobular carcinoma | 6 (3.9) |
Inflammation | 8 (2.5) | Atypical hyperplasia | 9 (5.8) |
Sclerotic lesions of the breast | 8 (2.5) | Lobular carcinoma in situ | 3 (1.9) |
Others | 3 (0.9) | Others | 6 (3.9) |
Values are presented as number (percentage).
Section discrepancy of 2D US in static images and dynamic videos
The BI-RADS classifications were evaluated by two sonographers based on the 2D characteristics of the lesions depicted in static images and dynamic videos ( Table 2 ). In our study, there were some lesions with section discrepancy ( Figure 2 ). Sectional differences were detected in 53 (11.3%) lesions by static observation and in 50 (10.7%) lesions by dynamic observation. We found no statistically significant difference in the proportion of lesions with section discrepancy between dynamic and static observations (P > 0.05), but in the results of the two observation methods, the lesions with section discrepancy were incompletely matching.
Distribution of Breast Imaging and Reporting Data System Classification of All Lesions in Static Images and Dynamic Videos
A | |||||||||||||
---|---|---|---|---|---|---|---|---|---|---|---|---|---|
Static images | Dynamic videos | ||||||||||||
B | 3 | 4A | 4B | 4C | 5 | T | 3 | 4A | 4B | 4C | 5 | T | |
3 | 89 | 31 | 1 | 0 | 0 | 121 | 186 | 16 | 2 | 2 | 1 | 207 | |
4A | 21 | 136 | 7 | 2 | 1 | 167 | 17 | 61 | 6 | 4 | 2 | 90 | |
4B | 0 | 9 | 56 | 7 | 1 | 73 | 5 | 12 | 26 | 10 | 0 | 53 | |
4C | 0 | 4 | 10 | 53 | 7 | 74 | 7 | 2 | 8 | 53 | 9 | 79 | |
5 | 0 | 0 | 3 | 8 | 22 | 33 | 0 | 1 | 0 | 14 | 24 | 39 | |
T | 110 | 180 | 77 | 70 | 31 | 468 | 215 | 92 | 42 | 83 | 36 | 468 |
A represents the horizontal plane, and B represents the sagittal plane.
Bold font represents the opposite classification nature.

A hypoechoic lesion located at 10’o clock in the left breast of a 25-year-old woman (A) Plane horizonal: the lesion is irregular and microlobulated (as pointed by the arrow), categorized as BIRADS 4C. (B) Plane sagittal: the lesion is oval and smooth, categorized as BIRADS 3. The final pathology was fibrocystic disease.
Correlation between the section discrepancy and histopathology
Either in static images or by dynamic videos, benign lesions were more likely to show section discrepancy than malignant lesions (P < 0.05). As presented in Figure 3 , the probabilities of section discrepancy in fibroadenoma, fibrocystic breast disease, and intraductal papilloma were significantly higher than other pathological types (P < 0.05). In malignant lesions, DCIS was more likely to show section discrepancy than other pathological types (P < 0.05). Finally, a value of 0.2 (P < 0.05) was obtained when the contingency coefficient was calculated for the correlation between section discrepancy and histopathology either in dynamic or static observation.
Sonographic features differences between static images versus dynamic videos
The sonographic features of 468 lesions assessed both in static images and dynamic videos are summarized in Tables 3 and 4 . Duct changes were more clearly depicted in dynamic videos than in static images (P < 0.05) both in malignant and benign lesions. Calcification and architectural distortion were more sensitively detected by dynamic videos than by static images (P < 0.05) in malignant lesions ( Figures 4 and 5 ). The interpretation of “margin” significantly differed in benign lesions between static images and dynamic videos (P < 0.05). More benign lesions displayed smooth edge in dynamic videos. On the contrary, they were more easily characterized as microlobulated or angular in static images ( Figure 6 ).
Sonographic Features of Benign Lesions (static images versus dynamic videos)
Ultrasonic characteristics | Benign (n = 315) | ||||||
---|---|---|---|---|---|---|---|
Static A | Dynamic A | P | Static B | Dynamic B | P | ||
Shape | Oval | 246 | 259 | 0.426 | 235 | 248 | 0.487 |
Circular | 18 | 14 | 28 | 24 | |||
Irregular | 51 | 42 | 52 | 43 | |||
Orientation | Parallel | 274 | 285 | 0.207 | 268 | 271 | 0.734 |
Non-parallel | 41 | 30 | 47 | 44 | |||
Margin | Smooth | 180 | 261 | 0.000* | 197 | 261 | 0.000 |
Microlobulated | 84 | 44 | 75 | 45 | |||
Angular | 9 | 5 | 9 | 2 | |||
Spiculated | 1 | 0 | 1 | 0 | |||
Indistinct | 44 | 8 | 36 | 8 | |||
Echo pattern | Hypoechoic | 293 | 280 | 0.340 | 289 | 288 | 0.876 |
Isoechoic | 2 | 5 | 3 | 3 | |||
Complexa | 6 | 9 | 5 | 8 | |||
Heterogeneousb | 14 | 21 | 18 | 16 | |||
Posterior features | Combined pattern | 7 | 6 | 0.880 | 7 | 8 | 0.942 |
Enhancement | 65 | 61 | 66 | 68 | |||
Shadowing | 0 | 0 | 1 | 2 | |||
Indifferent | 243 | 248 | 241 | 237 | |||
Calcifications | Not present | 295 | 287 | 0.323 | 297 | 283 | 0.061 |
Within lesion | 20 | 26 | 18 | 30 | |||
Outside lesion | 0 | 1 | 0 | 1 | |||
Intraductal | 0 | 1 | 0 | 1 | |||
Surrounding tissue changes | Architectural distortion | 2 | 2 | – | 2 | 2 | – |
Duct changes | 40 | 94 | 0.000 | 40 | 92 | 0.000 | |
Edema | 0 | 0 | – | 0 | 0 | – | |
Skin thickening | 3 | 3 | – | 2 | 3 | – | |
Skin retraction | 0 | 0 | – | 0 | 0 | – |
A represents the horizontal plane, and B represents the sagittal plane.
aContains both anechoic (cystic or fluid) and echogenic (solid) components.
bA mixture of echogenic patterns within a solid mass.
*P-values in bold face are statistically different; “–” represents not applicable.
Sonographic Features of Malignant Lesions (Static Images versus Dynamic Videos)
Ultrasonic characteristics | Malignant (n = 153) | ||||||
---|---|---|---|---|---|---|---|
Static A | Dynamic A | P | Static B | Dynamic B | P | ||
Shape | Oval | 50 | 49 | 0.683 | 41 | 41 | 0.979 |
Circular | 15 | 11 | 14 | 13 | |||
Irregular | 88 | 93 | 98 | 99 | |||
Orientation | Parallel | 107 | 111 | 0.613 | 102 | 104 | 0.807 |
Non-parallel | 46 | 42 | 51 | 49 | |||
Margin | Smooth | 29 | 41 | 0.549 | 18 | 31 | 0.076 |
Microlobulated | 92 | 98 | 90 | 102 | |||
Angular | 13 | 17 | 12 | 18 | |||
Spiculated | 3 | 3 | 1 | 5 | |||
Indistinct | 45 | 37 | 54 | 41 | |||
Echo pattern | Hypoechoic | 132 | 133 | 0.723 | 133 | 132 | 0.830 |
Isoechoic | 0 | 0 | 0 | 0 | |||
Complexa | 5 | 7 | 5 | 7 | |||
Heterogeneousb | 16 | 13 | 15 | 14 | |||
Posterior features | Combined pattern | 7 | 13 | 0.508 | 11 | 13 | 0.740 |
Enhancement | 38 | 40 | 48 | 43 | |||
Shadowing | 8 | 6 | 4 | 7 | |||
Indifferent | 100 | 94 | 90 | 90 | |||
Calcifications | Not present | 134 | 117 | 0.011 | 135 | 119 | 0.015 |
Within lesion | 19 | 36 | 18 | 34 | |||
Outside lesion | 0 | 0 | 0 | 0 | |||
Intraductal | 0 | 0 | 0 | 0 | |||
Surrounding tissue changes | Architectural distortion | 9 | 22 | 0.014 | 12 | 24 | 0.033 |
Duct changes | 15 | 64 | 0.000* | 22 | 59 | 0.000 | |
Edema | 0 | 0 | – | 0 | 0 | – | |
Skin thickening | 2 | 2 | – | 3 | 2 | – | |
Skin retraction | 0 | 0 | – | 0 | 0 | – |
A represents the horizontal plane, and B represents the sagittal plane.
*P-values in bold: represents statistically different; “–” represents not applicable.
aContains both anechoic (cystic or fluid) and echogenic (solid) components.
bA mixture of echogenic patterns within a solid mass.

(A) A hypoechoic lesion at 10’o clock in the right breast of a 44-year-old woman. (B) On static observation, the edges of the lesion appear to be either in the blue box or in the red box, making it difficult to define the presence of “calcification” (as shown by arrow) inside or outside the lesion.
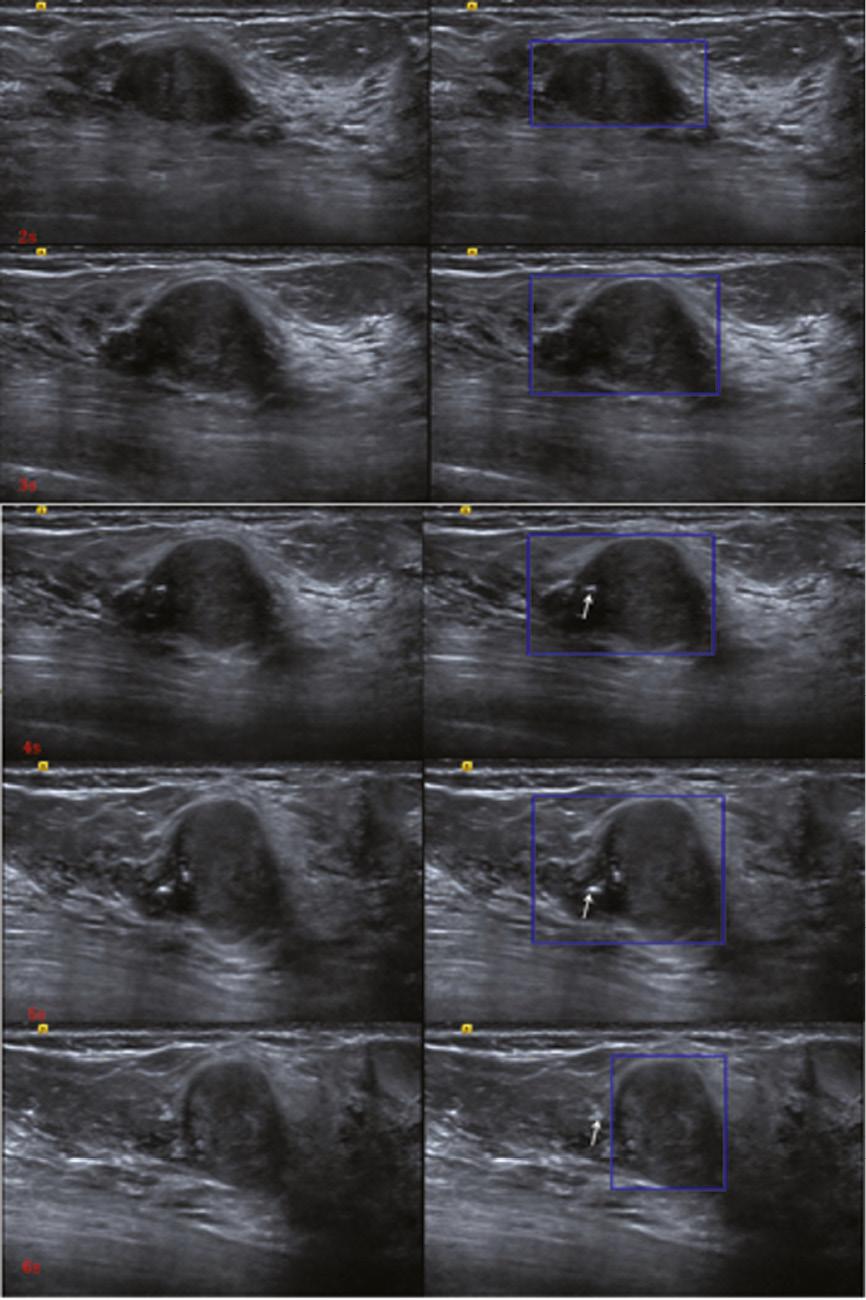
The time point screenshot of the dynamic video of the lesion shown in Figure 4 . With the scanning of the probe, the shape, orientation and edge of the lesion were changing (as shown in the blue box), and calcification was observed both inside and outside the lesion (as shown by the arrow).
Diagnostic performance of breast lesions at 2D US by dynamic videos versus static images
The AUC-ROC of dynamic videos were slightly higher than those of the static images ( Figure S1 ). The AUC values of static image-horizontal, static image-sagittal, dynamic video-horizontal, and dynamic video-sagittal were 0.807, 0.820, 0.837, and 0.846, respectively ( Table 5 ). Only the AUC values of static image-horizontal and dynamic videos-horizontal were significantly different (P = 0.026). As for specificity and accuracy, dynamic videos were higher than those of static images (P < 0.05). Dynamic observation had a similar sensitivity to static observation (P > 0.05). The consistency of dynamic and static observations between two sonographers had been showed in Supplementary Table 1 .
Diagnostic Performance of 2D Ultrasound According to the BI-RADS® Categories
AUC | 95% Confidence interval | Sensitivity (%) | Specificity (%) | Accuracy (%) | PPV | NPV | |
---|---|---|---|---|---|---|---|
Static image A | 0.807 | 0.769–0.842 | 96.7 | 33.3 | 54.1 | 41.0 | 95.0 |
Dynamic video A | 0.837a | 0.801–0.870 | 88.9 | 62.8a | 71.4a | 54.0 | 92.0 |
Static image B | 0.820 | 0.782–0.854 | 96.7 | 36.8 | 56.4 | 43.0 | 96.0 |
Dynamic video B | 0.846 | 0.810–0.878 | 90.2 | 60.9a | 70.5a | 53.0 | 92.0 |
A represents the horizontal plane, and B represents the sagittal plane.
aThe diagnostic efficacies based on static images and dynamic videos were statistically different.
BI-RADS, Breast Imaging and Reporting Data System; AUC, area under the curve; PPV, positive predictive value; NPV, negative predictive value.
Effects of breast tissue composition, lesion size, and depth on two observation methods
Based on breast tissue composition, the background echotextures were divided into fat type, fibroglandular type, and heterogeneous type. As shown in Figure S2 , compared with the consistent and inconsistent groups of dynamic and static observation results, there was no significant difference among the different background echotextures (P > 0.05). There was significant difference in lesion sizes between the two groups (P < 0.05). Most of the lesions in the inconsistent group were around 10 mm in the maximal diameter. As for lesion depth, there was no significant effect on the consistency of the dynamic and static observations (P > 0.05).
Discussion
Most atypical lesions appeared different ultrasonographic features with probe direction variation. The interpretation of ultrasonographic features was mainly based on typical images collected by sonographers. The selection of representative static images affected the final category of the lesions. Therefore, we investigated the section discrepancy and diagnostic performance of breast lesions in 2D US by dynamic videos versus static images.
Our research showed that section discrepancy of breast lesions existed in 2D US either by dynamic videos or in static images. We suspected that this may be because the section discrepancy is related to the limitations of 2D imaging, the subjectivity of the observer, and the pathological characteristics of the lesion itself. There was no obvious relationship between section discrepancy and the two observation methods. Our results confirmed that it was weakly correlated with histopathology. In previous study of breast elastography, section anisotropy was found to be highly correlated with malignant lesions. It was attributed to the growth pattern that oriented along the ducts [13, 14]. On the contrary, in our study, benign lesions were more likely to show section discrepancy than malignant lesions, whether in static images or in dynamic videos. This indicated that benign lesions were more likely to have both “benign” and “suspicious malignant” sonograms. In general, the occurrence and development of disease were a gradual process. In the process of occurrence and progression, the pathological features of the lesion were gradually manifested, and different stages might coexist. These different components of the lesion had different pathological characteristics [23], which contributed to the diverse ultrasonographic features of same pathological type.
In the study of Földi et al., statistically significant differences were observed regarding the interpretation of the “margin” of breast lesions between the video sequence and the freeze image [11]. However, statistically significant differences between dynamic videos and static images for the interpretation of the feature “margin” only existed in the benign lesion group of our study. In our study, most benign lesions were defined as “microlobulated” or “angular” in static images. The low contrast between the lesion and its surrounding tissue might have obscured the “margin” especially when the benign lesion did not have an intact or clear capsule. The ducts adjacent to the lesions were usually misinterpreted as part of the lesions in static images, which leads to a characterization of “indistinct margin.” However, with dynamic videos, the course of the ducts could be observed more clearly and be separated from the lesions. “Margin” is an important 2D feature that might affect the final impression of breast lesions. Focusing only on the static image might be the cause for the relatively low specificity of 2D US. We found that dynamic observation could obtain more complete and accurate information about lesions and surrounding relevant features, which would influence the final assessment of the lesions. Therefore, for the computer-aided diagnosis (CAD) system, which had been an active research field for tumor diagnosis owing to its operator-independent in terms of image acquisition and interpretation [24, 25], the prediction models based on the “training” data of static 2D US images may be biased. As is known to all, the CAD system involves the following four steps: image preprocessing, segmentation, feature extraction and selection, and classification [26]. Among them, image segmentation is a key step. In most situations, the segmentation of breast lesions was still performed by manually tracing the lesion contours in static images, which is time-consuming and experience-dependent. According to the results of our study, this traditional segmentation might be affected by the limitations of static US imaging, such as imaging angle, artificial selection, etc. [27, 28]. In the process of improving the segmentation accuracy, proposing automatic and real-time segmentation methods would be a main trend in this field [29–31].
In practice, we had found a large number of atypical lesions that were initially evaluated as BI-RADS 4 were pathologically benign. Our observation based on dynamic videos showed its superiority in differentiating atypical lesions, inconsistent with the study of Földi et al. [11]. This could be due to the smaller sample size (n = 45) in the study of Földi et al., with 30 benign lesions and 15 malignant lesions, leading to insignificant proportion of atypical lesions. Dynamic videos offered better observation of the whole breast lesion, not only the lesion itself, but also the relationship with the surrounding tissue. Our study results showed that the diagnostic performance by dynamic videos surpassed that of static images, which encouraged clinicians to develop CAD system based on dynamic US, since the source of representative and high-quality US was the premise of CAD systems research [32], which would increase the specificity of 2D US to avoid unnecessary waste of medical resources. If artificial intelligence could directly process dynamic videos, it could save time for manual tracing and avoid bias caused by different observers.
In terms of our study, the CAD system based only on 2D US might produce diagnostic bias because of section discrepancy. As we know, the automated breast US system (ABUS) has the capability to overcome some of these limitations by allowing structured image acquisition, which enables whole-breast evaluation with multiplanar reformation and temporal comparisons [33]. However, research on CAD-assisted diagnosis of ABUS is currently based on static images of lesions [34–35]. Therefore, even though the ABUS images have sufficient structural information, the gray contrast between the lesion and its surrounding tissue could still be similar to that of static 2D US.
Our study had several limitations. First, it was a retrospective single-center study, which might elicit selection bias. Second, patients who did not undergo surgery or biopsy were not included in the study, which, again, might cause selection bias. Third, the images and dynamic videos were all collected by one experienced sonographer; therefore, whether the image-collecting process might affect the interpretation of the lesions characteristics could not be evaluated, which is important for further CAD analysis.