INTRODUCTION
Intellectual disability (ID) is a condition that hinders a person’s ability to acquire knowledge and perform daily activities. The condition can arise from various factors such as birth defects, infections, genetic conditions, and injuries. Children who have intellectual disabilities may find it challenging to speak, walk, dress themselves, or eat without aid. They may also struggle with comprehending social cues and making friends. Figure 1 shows the flow of the thinking process. ID may be related to one or more components stated in the referenced diagram.
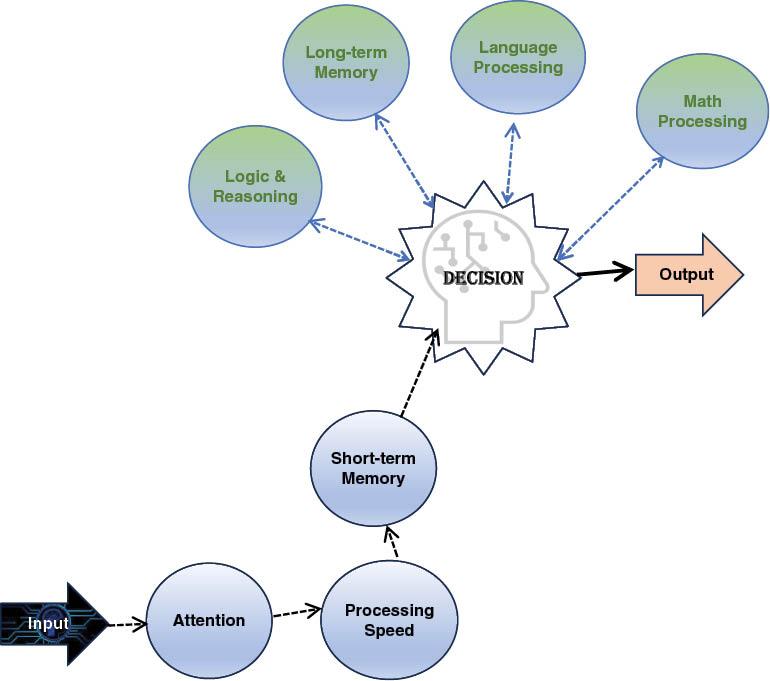
Thinking flow diagram: input to automatic processing group (attention, processing speed, short-term memory), leading to a higher thinking group.
Though there is no known cure for ID, there are numerous treatments available that aid the people with the condition in living a full and productive life. Early intervention is vital, and children diagnosed with intellectual disabilities should be given the opportunity to receive specialized education and support services.
With adequate support, individuals with intellectual disabilities can lead fulfilling lives. They can secure jobs, get married, and have their own families. Identifying the severity of ID in young children can be challenging, but there are certain indications that may point to an issue. Some symptoms that children with ID may exhibit include delayed achievement of developmental milestones such as crawling, sitting up, or walking, difficulty with speech and communication, difficulty remembering things, trouble grasping social norms, struggling to understand the consequences of their actions, and difficulty solving problems. ID pertains to a range of impairments in cognitive abilities that hinder functioning in two primary areas: intellectual functioning, which involves skills related to learning, problem-solving, and decision-making, and adaptive functioning, which encompasses activities such as communication and independent living that are necessary for daily life. Additionally, these limitations in intellectual and adaptive functioning are often noticeable in early childhood.
This paper targets to emphasize the magnitude of involvements and assistance systems, the responsibility of artificial intelligence (AI) in refining disability diagnosis, and the larger impression of AI expertise on authorizing and refining the excellence of life for persons with disabilities. The fundamental aim is to add to a more comprehensive and handy society where people with disabilities can live completing lives. This paper yields a contribution by suggesting a thorough assessment on the detailed attributes regarding frailties and AI. It suggests a thorough estimation of the existing involvements and support systems designed to help people with disabilities. Furthermore, it examines into the growth of AI frameworks for diagnosing disabilities, explaining how cutting-edge technology can enhance the appropriate identification and handling of disabilities. Likewise, it argues the larger role of AI in increasing the overall well-being of people with disabilities, including accessibility, education, and employment opportunities. This paper asks for closing the gap connecting present-day methodologies and emerging technologies, with the objective of founding a more widespread and helpful ecosystem for individuals with disabilities.
The primary research objectives of this paper are as follows:
To inspect and present a thorough overview of the existing involvements and support systems for people with disabilities.
To investigate the expansion of the AI framework for disability diagnosis and gauge its potential impact on early detection.
To explore the comprehensive role of AI in enhancing the lives of people with disabilities, including approachability, education, and social annexation.
To support to the current dialogue on disability sustenance and AI incorporation, with the goal of advancing a more inclusive and accessible society.
INTERVENTION AND SUPPORT
The early detection of ID is essential for implementing effective treatment and management strategies. This enables healthcare professionals to promptly administer appropriate interventions and support services tailored to the individual’s specific requirements. Timely interventions, including speech therapy, occupational therapy, and specialized educational programs, contribute to the enhancement of cognitive, language, social, and adaptive skills. Moreover, such programs facilitate the identification of any underlying medical or genetic factors that may contribute to the manifestation of ID. Through timely diagnosis and intervention, individuals with ID can increase their likelihood of realizing their full potential and leading gratifying lives.
Causes and diagnosis
ID is a neurodevelopmental condition characterized by impairments in both intellectual and adaptive functioning. Intellectual functioning pertains to the cognitive capacities encompassing learning, reasoning, and problem-solving abilities. On the other hand, adaptive functioning encompasses an individual’s proficiency in executing everyday tasks, including self-care, communication, and social skills.
Psychometric tests are used to assess intellectual functioning. These tests measure a person’s cognitive abilities, such as their memory, attention, and problem-solving skills. While there is no single intelligence quotient (IQ) score that definitively indicates ID, a full-scale IQ score of 70 to 75 is generally considered to be a significant limitation in intellectual functioning.
Nevertheless, it is crucial to take into account the comprehensive cognitive capabilities of the individual and avoid exclusively depending on the IQ score as an absolute metric. Variability in subtest scores can lead to the full-scale IQ score failing to precisely represent the individual’s overall intellectual functioning. Consequently, the interpretation of IQ test results necessitates the application of clinical judgment.
ID is characterized by challenges in both intellectual and adaptive functioning. To assess intellectual functioning, comprehensive and culturally appropriate psychometric tests of intelligence are employed. Although a specific full-scale IQ score is no longer a mandatory criterion for diagnosis, standardized testing remains a crucial component of the diagnostic process. Individuals with a full-scale IQ score of approximately 70 to 75 may demonstrate significant limitations in intellectual functioning, but this representation could be distorted due to variations in subtest scores. Hence, clinical judgment plays a vital role in the accurate interpretation of IQ test results.
Adaptive functioning is evaluated across three domains: conceptual, social, and practical. Conceptual domain involves language, reading, writing, math, reasoning, knowledge, and memory. Social domain encompasses empathy, social judgment, communication skills, adherence to rules, and the ability to establish and maintain friendships. Practical domain involves independence in areas such as personal care, job responsibilities, money management, recreation, and organizing tasks related to school and work.
ID can be classified into mild, moderate, or severe categories. Among these, mild is the most common. Symptoms of ID typically get definite during childhood, resulting in delays in language or motor skills. These symptoms are observable as early as two years of age. However, mild ID may not be diagnosed until a child reaches school age, when academic difficulties become more noticeable.
ID can have diverse underlying causes. Down syndrome or Fragile X syndrome, examples of genetic syndromes, may be related. Additionally, it can be caused by diseases like meningitis or measles. Exposure to toxic substances like lead or mercury or childhood head trauma are also potential factors. Furthermore, ID may be associated with brain malformations, maternal diseases, and environmental influences, such as alcohol, drugs, or other toxins.
Treatment
ID constitutes a persistent condition necessitating continuous assistance for affected individuals to operate effectively in their day-to-day activities. The etiology of this disability may encompass medical or genetic factors, as well as concurrent conditions, further complicating the challenges encountered by those with intellectual disabilities.
Postdiagnosis, the emphasis transitions to the recognition of the individual’s aptitudes and requirements, and the provision of suitable support to facilitate efficient functioning in domestic, educational or occupational settings, as well as within the community. An extensive array of services exists to assist individuals with intellectual disabilities and their families in fully engaging within their communities. Such services comprise early intervention initiatives, specialized education, familial support programs, transitional services, vocational training, daytime programs, housing and residential alternatives, and case management for service coordination.
Pursuant to the Individuals with Disabilities Education Act (IDEA), federal legislation mandates the implementation of early intervention measures for neonates and young children grappling with disabilities, including ID. Furthermore, IDEA guarantees free access to specialized education and associated services for all qualifying children with intellectual disabilities.
Assistance for those with intellectual disabilities may emanate from a diversity of sources such as relatives, acquaintances, colleagues, community members, academic institutions, medical professionals, and service systems. Appropriate support enables individuals with intellectual disabilities to lead prosperous and fruitful lives.
AI FRAMEWORK FOR DIAGNOSIS OF DISABILITY
The proposed framework delineates a systematic methodology for imposing AI in the diagnostic process of disabilities. This strategy emphasizes the significance of data acquisition, comprehensive preprocessing, feature determination, model development, validation, explainability, execution, continuous monitoring, human–AI collaboration, ethical considerations, and compliance with regulations. Through the utilization of this all-inclusive framework, disability diagnosis can exhibit increased accuracy and expediency, ultimately augmenting the quality of life for individuals experiencing disabilities.
Figure 2 shows the steps involved in training a machine learning model for diagnosis. First, signals or images are input into the model. Then, the data are preprocessed to clean them. Next, features are extracted from the data and ranked to identify the most significant features. Finally, the data are classified into normal or abnormal classes.
Gathering and merging data
In this phase, the collection and integration of data from multiple sources with various modalities become a vital component of comprehensive disability diagnosis frameworks. The data acquisition process encompasses three primary sources:
Medical records
The gathering and combining of previous medical records form a basis of this phase. These documents comprise patient medical history, diagnostic examinations, and documentation of prior medications. By combining this information, healthcare professionals obtain significant understandings into a patient’s medical journey, which is necessary for truthful and complete disability diagnosis.
Sensor data
Collecting data from wearable sensors, smartphone applications, and Internet of things devices performs a fundamental job in realizing a patient’s physical and physiological actions. This real-time data specify necessary information, granting the disability diagnosis framework to display a patient’s health and well-being continuously.
Imaging data
For visual evaluations, the employment of medical imaging procedures such as magnetic resonance imaging’s, X-rays, or computerized tomography scans is important. These imaging modalities present high-resolution graphical knowledge, which can be obligatory for diagnosing disabilities and trailing their progress.
Data preprocessing
The data preprocessing phase is a critical step in the development of robust disability diagnosis frameworks. It involves several essential tasks that are instrumental in ensuring the quality and reliability of the data used for subsequent analysis.
First, noise, outliers, and inconsistencies within the collected data need to be carefully addressed. Noise can stem from various sources, including sensor inaccuracies or data transmission errors, and must be filtered out to prevent it from introducing inaccuracies in the analysis. Outliers, which are data points that significantly deviate from the norm, can also distort the results and must be handled appropriately. Inconsistencies in data formatting or labeling can lead to misinterpretation and errors, necessitating data cleansing procedures to maintain data integrity.
Another crucial aspect of data preprocessing is the standardization of data formats and units. Healthcare data can come from diverse sources and may be recorded in varying formats or units of measurement. To ensure data compatibility and consistency, it is necessary to standardize these aspects, making it easier to compare and integrate data from different sources.
Furthermore, data preprocessing techniques can enhance the quality of datasets. Oversampling is employed to address class imbalance issues, where certain disability categories may have limited representation in the data. By artificially increasing the number of samples for underrepresented classes, the model’s performance becomes more balanced and accurate. Data synthesis techniques generate additional data points through methods like data augmentation, which is especially beneficial for image-based data. Adapting data to specific domains involves tailoring the data processing steps to the particular characteristics and requirements of the disability under investigation.
Feature extraction
In the realm of disability diagnosis, the feature extraction phase is pivotal as it involves the identification and extraction of crucial information from various data sources. Physiological signals and sensor data provide rich insights into a patient’s health. For instance, heart rate variability can reveal valuable information about cardiac health, gait analysis can shed light on mobility issues, and patterns in muscle activity can indicate neuromuscular conditions. These relevant aspects are extracted and transformed into meaningful features, which can serve as inputs for AI models, facilitating the diagnostic process. Disability diagnosis frameworks employ multimodal data, encompassing medical images and textual information, to furnish comprehensive and precise evaluations. Through computer vision methodologies, pertinent features are extracted from images, while natural language processing (NLP) techniques discern vital details from text. The amalgamation of these disparate data sources facilitates a comprehensive perspective on a patient’s health and medical history, connecting physiological, imaging, and textual data gaps. These extracted characteristics function as essential foundations for subsequent stages in the diagnostic process, empowering AI systems to identify patterns, make informed determinations, and ultimately contribute to enhanced precision and completeness in disability diagnosis.
Feature selection and ranking
In the realm of data analysis and machine learning, feature selection and ranking hold paramount importance for enhancing the efficacy and precision of predictive models. This procedure entails pinpointing and appraising the most pertinent attributes within a dataset, which proves vital for sieving through the copious features at disposal and isolating those with the most substantial bearing on model performance.
Predominant methods of feature selection and ranking encompass correlation analysis, mutual information, and dimensionality reduction.
Correlation analysis is instrumental in discerning connections among distinct features, enabling the recognition of highly correlated pairs. Mutual information quantifies the degree of interdependence between attributes and the target variable, shedding light on their informativeness. Dimensionality reduction approaches such as principal component analysis and linear discriminant analysis serve to mitigate dataset intricacy by transmuting it into a lower-dimensional space while retaining the most crucial information. The ultimate goal of feature selection and ranking is to streamline the data for subsequent modeling, thereby enhancing model interpretability, reducing overfitting, and improving predictive accuracy.
Machine learning and AI models
Machine learning and AI models are essential for developing more accurate and efficient medical diagnosis. These models are designed to analyze the vast amount of patient data available. The choice of algorithm depends on the disability or condition being diagnosed and the data characteristics.
Various machine learning algorithms are available to researchers and medical practitioners. The selection depends on the specific problem and data. Decision trees, for instance, are commonly used for their simplicity and interpretability, making them suitable for situations where it’s important to understand the reasoning behind a diagnosis. Support vector machines excel at classifying data into different categories, making them valuable for conditions that exhibit clear patterns or separations. Utilizing deep learning, centered around neural networks, has proven beneficial for intricate and unstructured data types, encompassing medical imaging and textual information. Furthermore, ensemble techniques such as random forests or gradient boosting amalgamate multiple algorithms to enhance overall accuracy and resilience.
Machine learning and AI models serve a paramount function in bolstering the capabilities of healthcare experts, facilitating the prompt and precise identification of conditions, which ultimately culminates in improved patient prognosis and tailored treatment strategies. Choosing the suitable algorithm is an essential determination due to its direct impact on the model’s efficacy in gleaning valuable insights from medical datasets and delivering trustworthy diagnostic conclusions.
Validation and evaluation
Within the domain of machine learning and data science, the validation and evaluation phase holds significant importance in determining the efficacy and dependability of the established models. To accomplish this task, the dataset is commonly divided into three distinct segments: training, validation, and testing sets. This partitioning method aids in evaluating the model’s capability to generalize across novel, unencountered data.
Model performance is assessed through a range of performance measures, including accuracy, precision, recall, the F1 score, and the area under the receiver operating characteristic curve. These metrics offer valuable insights into various facets of the model’s performance, including its aptitude for making precise predictions, its ability to minimize erroneous positive and negative results, and its overall equilibrium between precision and recall.
In order to enhance model resilience and mitigate overfitting, strategies such as cross-validation are frequently utilized. This entails systematically dividing the data into distinct training and validation subsets to evaluate the model’s stability and efficacy under diverse circumstances. Fine-tuning hyperparameters represents another critical aspect of this stage, as it necessitates optimizing the model’s parameters to attain optimal performance with respect to the given data.
Interpretability and explainability
In healthcare applications of AI, ensuring the interpretability and explainability of model decisions is of paramount importance. This is especially crucial because clinical professionals need to not only trust but also fully comprehend the basis for the AI-generated results. The opacity of deep learning models, for instance, can be a significant concern. Therefore, techniques like generating model explanations or using interpretable model architectures are essential to bridge this gap between advanced AI and clinical practitioners. These efforts help healthcare providers understand the rationale behind a particular diagnosis, treatment recommendation, or patient risk assessment, thereby enhancing the overall acceptance and utilization of AI technologies in clinical settings.
Furthermore, once the AI model has been developed with a strong focus on interpretability and explainability, it can be integrated into healthcare systems and platforms, such as telemedicine applications and mobile health apps. These integrations extend the reach of AI-driven healthcare solutions, making them accessible to healthcare providers, caregivers, and even patients themselves. However, it’s imperative to ensure that such integrations adhere to strict healthcare regulations, including data privacy and security standards, such as Health Insurance Portability and Accountability Act (HIPAA) in the United States. This not only safeguards sensitive patient information but also upholds the ethical and legal responsibilities within the healthcare sector.
Continuous monitoring and feedback
AI applications in healthcare are not simply deployed and then forgotten. Instead, they are continuously monitored and updated to ensure that they remain effective and reliable in real-world clinical environments.
Continual assessment entails consistent evaluation of the model’s performance, precision, and adaptability to shifting patient populations and medical practices. This process assists in detecting and resolving performance degradation or unintended biases swiftly.
Feedback cycles are implemented to encourage constant learning and enhancement. These cycles consist of collecting new data and patient outcomes from clinical environments and incorporating this information into the model. This uninterrupted data flow enables the model to adjust, progress, and fine-tune its predictions based on actual patient encounters.
Consequently, the model remains current and persistently augments its diagnostic and prognostic capabilities, ultimately boosting patient care quality. This feedback-centric strategy is crucial for maintaining the AI system as a reliable and secure resource for healthcare professionals, rendering it an adaptable and dynamic aspect of the medical decision-making procedure.
Cooperation between AI and humans
It is critical for AI systems and medical professionals to work together in order to fully leverage the capabilities of AI-driven healthcare innovations. Within the diagnostic domain, fostering relationships where AI acts as a supplementary resource, rather than a substitute, for medical professionals’ expertise is essential. AI technologies can efficiently operate as decision-support mechanisms, assisting physicians and clinicians in making more accurate and expedited diagnostic conclusions.
This cooperative strategy guarantees that both AI and human experts can utilize their distinct advantages to enhance patient care. It also enables healthcare providers to develop trust in and adopt AI technology as a beneficial asset within their diagnostic processes.
To enable effective cooperation, it is crucial to supply resources and platforms that facilitate interaction and validation of AI-generated diagnosis among healthcare practitioners. These resources may encompass user-friendly interfaces that enable medical professionals to liaise with AI models, comprehend their decision-making methodologies, and contribute or authenticate using their clinical acumen and proficiency.
These platforms additionally function as tools for verification and oversight, guaranteeing that AI systems maintain a track record of producing dependable outcomes that meet the established benchmarks and norms within the healthcare sector. In summary, the partnership between AI and human healthcare professionals represents a collaborative synergy with the capacity to transform the diagnostic procedure, resulting in greater precision, efficiency, and patient-centered healthcare.
Ethical aspects
Ethical considerations play a pivotal role in the integration of AI in healthcare. To ensure the responsible development and deployment of AI technologies, several key ethical aspects need to be addressed.
First and foremost, obtaining informed and transparent patient consent is paramount. Patients must be fully aware of how their data will be used in AI-driven healthcare applications and what potential risks and benefits are involved; they should have the autonomy to grant or deny permission for their data to be utilized in this context. Respecting patient consent not only upholds individual rights but also fosters trust and confidence in AI systems within the medical community.
Maintaining patient privacy is another crucial ethical dimension. Healthcare data are highly sensitive, and it is essential that robust security measures are in place to safeguard this information against unauthorized access or breaches. Compliance with data protection regulations such as the HIPAA in the United States or the General Data Protection Regulation (GDPR) in Europe is vital to uphold patient privacy rights.
Moreover, addressing bias and promoting fairness is imperative in AI systems. AI algorithms can inadvertently perpetuate biases present in the training data, potentially resulting in unfair or discriminatory outcomes. Developing and employing techniques to detect and mitigate biases, as well as ensuring fairness in AI models, is essential to prevent harm and inequities in healthcare decision-making. Ethical considerations in AI for healthcare are a complex, ongoing process that requires a multi-dimensional approach to uphold both the potential benefits and ethical responsibilities associated with these technologies.
Compliance with regulations
Compliance with healthcare regulations is a fundamental requirement when implementing AI-driven disability diagnosis frameworks. These regulations are in place to ensure patient safety, data privacy, and ethical use of healthcare technologies. In the United States, the HIPAA sets stringent guidelines for the protection and confidentiality of patient data. HIPAA mandates that healthcare organizations and related technology providers maintain strict security measures, ensure patient data privacy, and limit access to sensitive medical information. Adhering to HIPAA is not only a legal requirement but also an ethical obligation to safeguard patient interests.
Similarly, in the European Union (EU), the GDPR establishes comprehensive rules regarding data protection and privacy, with specific provisions related to healthcare data. GDPR imposes strict requirements for the lawful processing of health data, necessitating consent, transparency, and data minimization. Compliance with GDPR is vital for AI-based disability diagnosis frameworks operating in the EU to respect patients’ rights and maintain ethical data handling practices.
In essence, ensuring compliance with these healthcare regulations is not only a legal obligation but also a critical aspect of responsible and ethical healthcare technology development. It safeguards patient privacy, fosters trust in AI-driven diagnostic systems, and promotes ethical data handling practices, all of which are essential for the successful integration of AI in healthcare.
ROLE OF AI IN PERSONS WITH DISABILITIES
Advancements in AI technologies offer a significant opportunity to transform the lives of individuals with disabilities. While improving the lives of individuals with disabilities is a primary objective of AI systems, these systems also have the potential to alleviate various disabling conditions. The American Association of Intellectual and Developmental Disabilities highlights the importance of evaluating individuals with intellectual disabilities to identify and implement appropriate supports and services that facilitate their thriving in the community throughout their lives.
Physical disabilities and AI
AI is rapidly advancing and is being utilized in multiple ways to enhance the quality of life for individuals with disabilities. There are various ways in which AI is being implemented to aid deaf, mute, and specially-abled individuals. Real-time captioning technology harnesses AI to generate captions for live audio, which can greatly benefit deaf and hard-of-hearing individuals in various settings, such as classrooms, meetings, and concerts ( Kawas et al., 2016; Millett, 2021). Similarly, sign language translation can convert sign language into text or speech, enabling deaf and hard-of-hearing individuals to communicate with those who do not comprehend sign language ( Camgoz et al., 2018; Guo et al., 2018).
AI is being used to develop robots that can help disabled people with everyday tasks. These robots can provide support and companionship, enabling people with disabilities to live more independently. Virtual reality (VR) and augmented reality (AR) can also be used to make captivating experiences for disabled people. For example, VR can allow people with visual impairments to experience the world around them, and AR can help people with physical impairments to complete tasks that would be otherwise difficult or impossible ( Arvanitis et al., 2009; Tang et al., 2015).
Brain–computer interfaces (BCIs) are devices that can translate brain signals into commands that can be used to operate computers or other devices. This means that people with disabilities that prevent them from using traditional input methods, such as people with paralysis or severe speech impairments, can use BCIs to control their environment and communicate with others. The utilization of BCIs holds promise in empowering disabled individuals to assume command over their surroundings and facilitate communication with others ( Kinney-Lang et al., 2020; Cruz et al., 2021). Figure 3 shows the typical flow of brain–computer interaction for physically impaired individual.
Gene-editing technologies, such as CRISPR, have the potential to treat or prevent genetic disorders that cause disabilities. For example, CRISPR could be used to correct mutations in the genes that cause cystic fibrosis or muscular dystrophy ( Hasanzadeh et al., 2022).
AI-enhanced wheelchairs possess the ability to navigate obstacles, evade collisions, and ascend stairs proficiently ( Rahimunnisa et al., 2020). AI-powered prosthetic limbs can be controlled by thought, and can be customized to the individual user’s needs ( Elbreki et al., 2022). AI-powered eyeglasses can provide real-time captions for people with impairments, and can translate languages ( Sneha et al., 2022). AI-powered hearing aids can be programmed to filter out background noise and amplify speech ( Balling et al., 2021). AI-powered software can be used to assist disabled individuals with tasks such as writing, reading, and math.
Intellectual disability and AI
People who have intellectual disabilities face difficulties when trying to access and participate in community services because of obstacles such as communication barriers, lack of accessibility, cognitive hindrances, and sensory impairments. The aim of AI is to consistently provide solutions that help improve the support available to these individuals. Figure 4 shows different domains of ID, where AI can be helpful.
Diagnosis with AI
AI can be employed for the diagnosis of ID using a range of methods. Cognitive assessments, behavioral observations, and medical records can be analyzed by AI algorithms to detect patterns and indicators of ID ( Di Nuovo et al., 2014; Green and Flaro, 2016; Uddin et al., 2019). It makes AI systems to help medical professionals in examining persons for probable ID patients ( Kim et al., 2021).
AI can also be used to analyze brain imaging data, to identify structural abnormalities or irregularities that can be associated with ID. Machine learning algorithms are very helpful in finding patterns by training on large datasets of brain images. That can help to support the diagnosis of ID ( Abd-Alrazaq et al., 2022; Gupta et al., 2022; Sadeghi et al., 2022; Zhang et al., 2023). AI systems can also use textual data for diagnosis. NLP techniques are used to analyze written or spoken language. This can help to identify symptoms, history or distinct markers associated with ID ( de la Fuente Garcia et al., 2020; Le Glaz et al., 2021; Ratana et al., 2023). Such NLP based AI algorithms can also be used to analyze interviews or written assessments that can identify association with ID. AI-based systems can also be used to administer and analyze developmental assessments. By observing and analyzing a child’s behavior, responses, and interactions, AI algorithms can help to identify developmental delays or signs of ID ( Tariq et al., 2019). Furthermore, the utilization of AI extends to the examination of genetic data, such as DNA sequencing, aiming to discern genetic variations or mutations linked to ID. Machine learning algorithms assume a crucial role in discerning patterns within genetic data, consequently augmenting the diagnostic capabilities for ID ( Quinodoz et al., 2017; Movaghar et al., 2021; Donnelly et al., 2023).
AI-based therapies
AI is currently harnessed to craft individualized therapies for individuals with intellectual disabilities. These cutting-edge therapies leverage AI algorithms to meticulously scrutinize data encompassing cognitive abilities, behavioral patterns, and unique characteristics of the individual. Subsequently, this data-driven analysis forms the foundation for creating personalized intervention plans, meticulously designed to address and cater to the specific needs of each individual ( Benrimoh et al., 2018). This personalized approach tailors therapy programs to the unique needs and abilities of each individual, maximizing their potential for growth and development.
Adaptive learning environments can be facilitated by AI to benefit individuals with intellectual disabilities. Through continuous monitoring and assessment, AI algorithms can dynamically adjust the difficulty level and content of therapy sessions. This adaptive approach ensures that individuals are consistently challenged while maintaining an optimal learning pace that suits their abilities ( Di Nuovo et al., 2018; Salhi et al., 2022).
AI-based therapies also play a significant role in improving speech and language skills for individuals with intellectual disabilities ( Sharma and Singh, 2022). Combining speech recognition technology with NLP, AI systems provide real-time feedback, interactive communication tools, and practice exercises. These features enable individuals with ID to enhance their communication abilities, fostering more effective social interactions ( Myszczynska et al., 2020).
Addressing emotional and social challenges is another area where AI-based therapies prove valuable for individuals with intellectual disabilities. By employing facial recognition technology, AI systems can identify and interpret emotions, aiding individuals with ID in understanding and expressing their own feelings ( Ghafghazi et al., 2021). Moreover, this technology enhances their ability to recognize emotions in others. VR and avatar-based interactions can further simulate real-life social scenarios, fostering the improvement of social skills and promoting social integration ( Leghari and Syed, 2023).
One significant advantage of AI-based therapies is their accessibility, particularly in remote settings. Individuals with intellectual disabilities, especially those residing in underserved areas or with limited access to specialized therapists, can benefit from AI-powered teletherapy platforms. These platforms enable remote sessions, offering convenient and consistent therapy support regardless of geographical location. AI-based therapies hold immense promise in revolutionizing the support and intervention received by individuals with intellectual disabilities. By harnessing the power of AI, these therapies provide personalized, adaptive, and accessible approaches to enhance cognitive, communication, emotional, and social skills. Ultimately, these advancements improve the overall quality of life for individuals with ID.
Personalized learning
Students diagnosed with intellectual disabilities frequently experience significant health concerns necessitating extra medical attention and supervision. These health issues contribute to higher rates of absenteeism and can impede academic progress and the development of adaptive and social skills. By integrating AI into the education of students with intellectual disabilities, it is possible to mitigate the educational, adaptive, and social skill gaps that arise due to persistent health problems. The goal of personalized learning for individuals with intellectual disabilities is to customize educational methods, resources, and support to fit their distinct needs, capabilities, and learning preferences. It acknowledges that every person with an ID possesses unique strengths, difficulties, and preferences that must be taken into account to enhance their learning experience. Personalized learning for intellectual disabilities centers on creating adaptable, individualized, and flexible educational programs. This entails evaluating the person’s present abilities, interests, and aspirations and formulating customized learning plans accordingly. These plans should cover customized curricula, assistive technologies, specific teaching techniques, and amendments ensuring maximum involvement and progress ( Grigal et al., 1997). AI can be employed to create personalized learning plans for people with ID. These plans should focus the specific needs and abilities of target person ( Hills et al., 2018). Studies published by Kharbat et al. (2021) highlights the fact that these AI learning platforms can be very helpful in enhancing the motivations of ID persons. The study found that students who used an AI-powered learning platform were more engaged in their educational activities and more motivated to learn than students who did not use the platform.
Number of research studies support that AI-based personalized learning can be beneficial for people with ID ( Nganji and Brayshaw, 2017; Akyuz, 2020; Zdravkova, 2023). These studies also suggest that AI can be used as an effective tool for personalized learning among students with intellectual disabilities.
Virtual assistants
Virtual assistants (VAs) can be a great help for people with physical and intellectual disabilities ( Lindholm et al., 2005). VAs have the capability to interact with intelligent devices within a domicile, thereby controlling the atmosphere, such as activating and deactivating illumination systems, obviating the need for physical actions. These assistants function as an essential support mechanism for individuals with injuries, as they enable communication with emergency responders or solicitation of aid. Furthermore, VAs exhibit significant compatibility with persons experiencing cognitive impairments. Progress in VA technology is fostering the creation of accessible user interfaces for all users, facilitating operations such as initiating telecommunications, transmitting messages to caregivers, disengaging access barriers, and coordinating grocery deliveries ( Canbek and Mutlu, 2016; van Wingerden et al., 2023). People with disabilities may find it difficult to communicate and coordinate with others. VAs can assist them in communication as well. VAs have been successful in supporting people with disabilities by automating tasks that require human intelligence and support. VAs can also be personalized to meet the specific needs of each individual.
Augmentative and alternative communication devices
Augmentative and alternative communication (AAC) devices, systems, strategies, and tools can be used by people who have difficulty communicating using speech. Augmentative communication refers to supplementing, enhancing or adding to someone’s speech. Alternative communication is used to replace the speech. AAC devices are very helpful for persons with intellectual disabilities ( Farzana et al., 2020; Li et al., 2022). Different types of symbols and written or spoken text can be produced by these devices. Not all tools are created equal, so it is important to find one that is tailored to your specific needs ( Park et al., 2022).
These AAC devices are significantly important for improvement of verbal communication ( Murero et al., 2020). There is a wide variety of these devices available in market. These includes text-based, symbol-based and Multimodal communicators. AAC devices help indicviduals to express their thoughts, ideas, desires, and needs in more effective way ( Elsahar et al., 2019; Costanzo et al., 2023).
AAC devices are primarily focused on language skills of ID personnel. These provide the visual representations of phrases, dictionary words, and varying concepts. Moreover, AAC devices also offer text-to-speech functionality, which proves especially beneficial for individuals facing challenges in reading and writing ( Ganz et al., 2017). AAC devices offer a multitude of advantages to individuals who experience challenges in verbal communication. These benefits encompass fostering stronger friendships and deeper relationships, facilitating more frequent and meaningful social interactions, enhancing social roles, empowering individuals with increased autonomy and decision-making capabilities, promoting greater independence, earning higher respect from others, encouraging active engagement in family and community activities, facilitating improved information exchange with healthcare professionals, enhancing personal safety, unlocking more employment and volunteer prospects, and contributing to enhanced physical and mental well-being ( Mcnaughton and Bryen, 2007; Chan et al., 2019).
AAC devices can help people with communication difficulties to build stronger relationships with their friends and family, have richer and more frequent social interactions, take on deeper social roles, have more autonomy and decision-making power, be more independent, be treated with more respect by others, participate more fully in their family lives and communities, share information with their physicians more effectively, be safer in a variety of settings, find more employment and volunteer opportunities, and have improved physical and mental health.
Robotic assistants
AI-powered robotic assistants can help people with intellectual disabilities with tasks such as cooking ( Sugiura et al., 2011; Bollini et al., 2013; Inagawa et al., 2021), cleaning ( Prabakaran et al., 2018), and getting around ( Wagemaker et al., 2017). Robots can offer companionship and support to people with intellectual disabilities, helping them to live more autonomously. Robots can provide a sense of companionship and social interaction, which can be advantageous for individuals with intellectual disabilities who may have trouble interacting with others ( Mitchell et al., 2021).
These illustrations represent only a fraction of how AI is being employed to aid individuals with intellectual disabilities. As AI technology advances, we can anticipate a plethora of innovative and beneficial applications catering to the specific needs of people with intellectual disabilities.
CONCLUSION
People with intellectual disabilities can benefit from AI technology in several ways. One significant advantage is increased independence in their daily lives. AI-powered home assistants can help people with intellectual disabilities manage their homes without having to move around. AI can also improve communication for people with intellectual disabilities. Speech-to-text software that uses AI can help people with speech impairments communicate verbally. Similarly, AI-powered sign language translation software can help deaf people communicate better with hearing people.
AI technology can play a pivotal role in fostering a sense of connection and reducing isolation among individuals with intellectual disabilities. Social media platforms leveraging AI can facilitate connections with others who share similar experiences, while AI-powered video chat software enables better communication and regular contact with friends and family.
In a broader sense, AI holds the potential to substantially enhance the quality of life for people with intellectual disabilities. By promoting increased independence, enhanced communication, and decreased isolation, AI can empower individuals with intellectual disabilities to lead more enriching and autonomous lives.