Introduction
In 2017, 40% of Irish rivers failed to meet the required status of “high” or “good” water quality as set out in the Water Framework Directive 2000/60/EC (WFD). In 2020 the Environmental Protection Agency (EPA) reported a further decline in water quality with 47% of rivers having unsatisfactory nitrate levels and 34% having unsatisfactory phosphorus levels. Findings also show geographical differences, and areas with intensive farming activity and free draining soils are more susceptible to losses (EPA, 2020). The European Commission has highlighted the agricultural sector as a particular challenge for Ireland in meeting water quality objectives (Commission, 2012) because Ireland has a larger livestock sector relative to most other European countries (Figure 1). Livestock produce manure; hence, larger livestock numbers coupled with a grass-based production system increase the risk of nitrate pollution to watercourses (Buckley et al., 2016; Sahoo et al., 2016).
In the most recent Farm Structural Survey (FSS) carried out by the Irish Central Statistics Office (CSO) in 2016, specialist beef production was the dominant type of farming on 57% of Irish farms. Farms that have some degree of livestock production account for 90% of the total number of farms in Ireland in 2016. The removal of European Union (EU) dairy quotas in 2015 resulted in an expansion in the Irish dairy herd, adding to the environmental pressures associated with livestock (Chen & Holden, 2018; Läpple & Sirr, 2019). An increase in livestock numbers implies increased demand for grass, which in turn implies an increase in demand for chemical fertilisers. Excessive applications of chemical fertilisers can result in increased runoff to water bodies causing diffuse pollution (Wu & Ma, 2015). However, in recent years, the national herd has remained relatively stable with falling suckler cow numbers balancing out some of the expansion on the dairy side (see Figure 2).
One area that has the potential to balance the nexus between water quality and agricultural production is the area of nutrient management planning. Strict management of nutrients (nitrogen [N] and phosphorus [P]) mainly on agricultural land reduces the risk of nutrient mobilisation in runoff pathways to water bodies (Buckley et al., 2015), can significantly improve the farm-level profitability (Buckley & Carney, 2013) and also improve water quality (Rao et al., 2009). However, the economic benefits associated with fertiliser use and increasing crop yield far outweigh the cost of the fertiliser. This may encourage the over-application of fertilisers and manures in many countries (Goulding et al., 2007).
The challenge for policy makers is to identify and encourage best practices which can protect both farm incomes and the environment (Godfray & Garnett, 2014; Westhoek et al., 2014; Zhang et al., 2015). Therefore, understanding the factors that influence farmer’s perception of, and willingness to accept or engage, with nutrient management best practice is important (Flett et al., 2004; Li et al., 2007; Tey & Brindal, 2012; Pierpaoli et al., 2013; Kelly et al., 2016; Kuehne et al., 2017; Naspetti et al., 2017).
Technology acceptance and adoption has been a major topic of concern for researchers and policy makers (Dwivedi et al., 2017). The research can be divided into two groups: ex-post research that adopts a utility-based methodology and ex-anti research that adopts and makes use of predictive models (Pierpaoli et al., 2013). In this paper, we examine if an ex-anti model, the Technology Acceptance Model (TAM) can predict the underlying socio psychological factors which are deemed to be important drivers of technology acceptance and adoption.
The TAM was designed to provide valid measurement scales for predicting user acceptance of computers (Davis, 1989). This seminal paper was based on earlier psychological theories of behaviour, the Theory of Reasoned Action (TRA) (Fishbein & Ajzen, 1977; Ajzen, 1985) and the Theory of Planned Behaviour (TPB) (Ajzen, 1991). These earlier theories of behaviour highlight the importance of attitude, subjective norms and behavioural control in determining the future intention to adopt. TPB was applied to investigate factors explaining adoption of soil erosion practices and identified attitude towards the practice to be the most important explanatory factor (Wauters et al., 2010). Farmer attitudes, subjective norms and behavioural control were also found to be significant predictors of intention to utilise soil test results (Daxini et al., 2018). While these studies focus entirely on the characteristics of the farmer, the attributes of the technologies may also influence a farmer’s decision to adopt, specifically the farmer’s prior perceptions of the technology.
The TAM focuses on perceptions which influence the attitudinal aspect of intention to use (Nysveen et al., 2005; Heerink et al., 2008; Fathema et al., 2015) (Figure 3) and outlines a methodology to measure individual perceptions around usefulness and ease of use of a technology (Davis, 1989). Flett et al. (2004) was the first paper to apply a TAM to agriculture and highlighted the importance of socio psychological factors as important drivers of technology acceptance and adoption. Recent studies continue to emphasise the importance of including socio economic and psychological factors in models to help predict farmer adoption behaviours (Carli et al., 2017; Adnan et al., 2019; Streletskaya et al., 2020). Usefulness and ease of use have been shown to be the important drivers of technology adoption and that prior perceptions influence the attitudinal aspect of the behavioural decision (Folorunso & Ogunseye, 2008; Kutter et al., 2011; Pierpaoli et al., 2013). A TAM was used to identify the important factors influencing the adoption of a mobile-based agricultural extension service and found the TAM variables to be reliable and valid constructs in predicting behavioural intention to use (Verma & Sinha, 2018). The TAM has also been used to predict the use of natural pest control in rice production (Sharifzadeh et al., 2017) and the use of integrated pest management in horticulture (Rezaei et al., 2020).
This research adds to the literature by investigating the role of farmer perceptions in influencing the attitude in relation to the usefulness and the ease of use of a nutrient management plan (NMP); a positive attitude will improve the probability of future intention to adopt.
Materials and methods
Description of a nutrient management plan
A NMP is an online tool, based on soil test results, designed to assess the current nutrient balance of Irish farms and devise a fertiliser management programme that will optimise soil fertility and ensure compliance with the limits set under EU nitrates regulations. The programme uses the latest in online mapping technology to produce a farmer-friendly NMP, along with colour-coded maps (Teagasc, 2014). A NMP consists of a number of documents generated by an online system, which provides a farmer with detailed field-specific information in relation to nutrient management best practice, known as the 4Rs.
The 4Rs provide a framework for achieving the goals of increasing profitability while protecting the environment by applying the Right fertiliser at the Right rate, in the Right place at the Right time. The online NMP incorporates data from a number of sources, including soil samples, stocking rates, farm size and concentrates fed to generate a map with field-specific fertiliser application advice. The pH levels of each field are also included, since the correct pH is an important consideration for the efficient uptake of nutrients and liming advice is provided based on pH levels (Teagasc, 2014).
Soil testing has been an accepted agricultural management practice for decades. It has contributed to the increased efficiency of agricultural production by providing a sound basis for lime, manure and fertiliser application (Sims et al., 2000; Wall & Plunkett, 2016). However, high rates of soil testing do not of themselves imply strict management and an efficient application of nutrients. Previous literature has highlighted the fact, that even though there is a high uptake of soil testing by Irish farmers, soil fertility levels were also dropping (Kelly et al., 2016; Wall et al., 2018). Therefore, a soil test alone, without a detailed plan of where, when and how much nutrients to apply at field level, cannot insure the optimum use of nutrients to protect soil fertility, and avoid over-application, which may result in runoff to water bodies.
Conceptual framework
The TAM is an ex-ante theoretical model based on the TRA that was specifically designed to develop key theoretical constructs to predict systems’ use (Davis, 1989). The TAM suggests that external influences directly and indirectly influence farmer beliefs and that there are two beliefs which can predict system acceptance and use, namely, perceived usefulness (PU) and perceived ease of use (PEOU) (Legris et al., 2003). PU is defined as “the degree to which a person believes that using a particular system would enhance his or her job performance” (Davis, 1989). PEOU is defined as “the degree to which a person believes that using a particular system would be free of effort” (Davis, 1989).
A key purpose of TAM is to provide a basis for tracing the impact of external variables on internal beliefs (PU and PEOU), which influence attitude towards use (A), behavioural intention to use (BI) and actual system use (Legris et al., 2003). Figure 1 outlines the importance of PU and PEOU in terms of attitude towards use (A) and the behavioural intention to use (BI) and actual use. According to the TAM, PU and PEOU are the main factors influencing an individual’s attitude which in turn influences their behavioural intention and actual use (Engle et al., 2010).
The TAM approach has also been applied in agricultural research to explain adoption and use of dairy farming technologies in New Zealand (Flett et al., 2004), to explain the difficulties of precision agriculture technology adoption in Canada (Aubert et al., 2012), to predict the intention to use six grassland management practices on specialist dairy farms in Ireland (Kelly et al., 2015) and to evaluate the main factors influencing grazing technology adoption among new entrant dairy farmers in Europe (McDonald et al., 2016). TAM is designed to elicit responses, from a user perspective, on their perceptions of using a technology ex-anti and is designed to capture and test the strength of unobservable or latent factors which influence the adoption decision.
In the context of this study, we consider if a TAM can provide ex-anti information on whether a farmer might adopt a NMP and which underlying latent factors influence the adoption decision. The importance of understanding the strength and significance of these unobservable factors can help knowledge transfer specialists in providing farmers with the information necessary to address the importance of the psychological factors regarding how useful and how easy to use the technology can be. Therefore, we hypothesise that if a farmer perceives a NMP to be useful and easy to use there is a higher likelihood of adoption.
Data
The data used in this paper were collected from farmers involved in a unique programme, the agricultural catchments programme (ACP) and a control group of farms with similar characteristics, not participating in the programme. The function of the ACP is to evaluate the environmental and economic effects of the Nitrates Directive (91/676/EEC, 1991). The ACP has been in place for over 10 yr. It is a multi-disciplinary team, involving researchers (a soil scientist, a hydrologist and an economist), knowledge transfer specialists (agricultural advisors), data technologists (data processing) and technicians (equipment upkeep and data collection). The ACP consists of six catchment areas, which were chosen as examples of intensive grassland and arable agriculture over varying soil types and with different considerations of phosphorus and nitrogen transfer risk (Fealy et al., 2010).
A questionnaire was designed to collect data from farmers across a range of topics, including nutrient management best practices (soil testing, NMP, application of lime, etc.). Questions in relation to the use of a NMP were specifically designed to elicit farmers’ opinion on the PU and PEOU of the technology. The survey was collected in 2015 by a team of professional recorders, on a total of 358 farmers.
In this paper, a two-step approach is applied, first the PU and PEOU are predicted using a factor analysis of the statements which are designed to capture the PU and the PEOU of using a NMP. Secondly, PU and PEOU are explanatory variables in an ordered logit regression on the farmer’s future intention to adopt, where the dependent variable is the statement “I intend to use a NMP for decision making in the next 12 months”.
The TAM model hypothesises that the latent factors PU and PEOU are a best proxy for farmer beliefs and that these beliefs are crucial to farmer attitudes towards future intention to use of a technology.
Perceived usefulness and perceived ease of use
Following the TAM methodology, survey questions were selected to assess the underlying PU and PEOU of a NMP (Table 1) where responses were scored from “strongly disagree” to “strongly agree” on a scale of 1–5 (strongly disagree, disagree, neutral, agree, strongly agree). Statements 1–5 are hypothesised to correspond to PU and statements 6–7 correspond to PEOU (i.e., we expect the factor loadings to clearly identify two separate factors based on these statements).
Survey questions to assess the underlying PU and PEOU for NMP
Statement | Strongly disagree | Disagree | Neutral | Agree | Strongly agree |
---|---|---|---|---|---|
1. NMP is important to my farming needs | 1 | 2 | 3 | 4 | 5 |
2. NMP is better than no plan | 1 | 2 | 3 | 4 | 5 |
3. NMP increases production | 1 | 2 | 3 | 4 | 5 |
4. NMP increases my profits | 1 | 2 | 3 | 4 | 5 |
5. NMP saves time | 1 | 2 | 3 | 4 | 5 |
6. NMP results are easy to understand | 1 | 2 | 3 | 4 | 5 |
7. NMP results are easy to use | 1 | 2 | 3 | 4 | 5 |
NMP = nutrient management plan; PU = perceived usefulness; PEOU = perceived ease of use.
Rational for statements to capture PU and PEOU
The first question (1) “A NMP is important to my farming needs” assesses the farmer’s perception of how the management practice fits the individual needs and objectives of their farm. The question is designed to account for heterogeneity across farming systems and farmer objectives. It relates to the overall perception of the use of the technology to a particular farming system and the characteristics of their farm. Question (2) “NMP is better than no NMP” captures the farmers PU of the technology in terms of relative advantage and whether the new technology is perceived to be an improvement on the existing practice. This is a key component of innovation adoption (Rogers, 2010). Question (3) “NMP increases production” and question (4) “NMP can increase my profits” capture the importance of financial benefits to the farm. These are a key motivation in terms of farmer use (Flett et al., 2004; Kelly et al., 2016). Time management is also considered to be an important consideration for many farmers (Kitchen, 2008; Vaarst & Sørensen, 2009), and therefore the fifth PU question (5) “NMP saves time” is included to assess the time management consideration in relation to use and adoption.
The factors that influence PEOU are associated with physical and mental effort which a farmer associates with the adoption and use of a technology as discussed above (Davis, 1989). Two questions were used to assess farmers’ PEOU of both technologies. These were question (6) “NMP results are easy to understand” and question (7) “NMP results are easy to use”. The PU and PEOU variables are latent constructs that emerge using a factor analysis of the statements in Table 1.
Ologit model
In this research, an ordered logit model is deemed the appropriate regression model, since the dependent variable is categorical and ordinal. The respondent strongly disagrees, disagrees, is neutral, agrees or strongly agrees with the statement “I plan to use a NMP for decision making in the next 12 months”. Since the order of these responses matters, and not just whether the respondent falls into the category or not, an ordered logit regression is appropriate.
The ordered logit model can be defined as:

where Y * is the unobserved dependent variable “intention to use a NMP for decision making in the next 12 months”, X are the independent latent variables PU and PEOU and ε is the error term.
Since farmers face j ordered alternatives with cut-off or threshold points a such that:
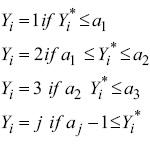
where
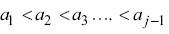
In this study, there are five j alternatives: strongly agree, agree, neutral, disagree, strongly disagree ordered 1–5; subsequently, there are four cut-off or threshold points a.
The probability that a farmer chooses j is defined by the odds ratio:
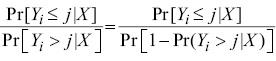
where
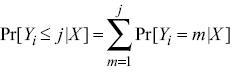

In this paper, a two-step approach is applied, first the PU and PEOU are predicted using a factor analysis of the statements which are designed to capture the PU and the PEOU of using a NMP. Secondly, PU and PEOU are explanatory variables in an ordered logit regression on the farmer’s future intention to adopt, where the dependent variable is the statement “I intend to use a NMP for decision making in the next 12 months”.
The TAM model hypothesises that the latent factors PU and PEOU are a best proxy for farmer beliefs and that these beliefs are crucial to farmer attitudes towards future intention to use of a technology. Table 2 provides the socio economic and demographic characteristics of the farmers who participated in the survey.
Descriptive statistics
Variable | Obs | Mean | s.d. | Min | Max |
---|---|---|---|---|---|
Age | 358 | 54.08 | 10.71 | 24 | 80 |
Married | 358 | 0.75 | 0.43 | 0 | 1 |
Years farming | 358 | 30.41 | 14.71 | 1 | 75 |
Successor identified | 358 | 0.45 | 0.50 | 0 | 1 |
Off-farm job | 358 | 0.23 | 0.42 | 0 | 1 |
Farm size (Ha) | 358 | 64.07 | 67.09 | 10.1 | 484.8 |
Share grassland | 355 | 0.81 | 0.33 | 0 | 1 |
Share tillage land | 355 | 0.18 | 0.32 | 0 | 1 |
Livestock system | 358 | 0.79 | 0.41 | 0 | 1 |
Tillage system | 358 | 0.14 | 0.35 | 0 | 1 |
Stocking rate (Lu Ha−1) | 318 | 1.59 | 0.78 | 0 | 4.455,445 |
Environmental scheme | 358 | 0.54 | 0.50 | 0 | 1 |
Extension services | 358 | 0.84 | 0.37 | 0 | 1 |
Has dairy | 358 | 0.27 | 0.45 | 0 | 1 |
Agricultural education | 358 | 0.60 | 0.60 | 0 | 2 |
Estimated gross income | 358 | 44,720.67 | 25,477.08 | 1,0000 | 100,000 |
Note: Farm Agricultural education includes any agricultural specific education up to and including an agricultural degree.
The farms who participated in the survey are a mix of tillage and livestock farms. Average farm size of 64 Ha is above average for Irish farms, and 26% of these farmers have off-farm employment. Therefore, almost 75% of these farmers would describe themselves as full-time farmers. Extension services relate to agricultural advisory services of which 84% of farmers have a farm advisor, and 60% have some formal agricultural education.
Results
Factor analysis
Factor analysis was carried out on the statements in Table 1 to capture the underlying farmer perceptions towards using a NMP. Factor analysis is typically applied to simplify complex sets of data. It operates on the principal that measurable and observable variables can be reduced to fewer latent variables or factors (Bartholomew et al., 2011). Factors were extracted based on factor loadings greater than 0.5. An oblique rotation was used to obtain simple structure, since the factors are known to be correlated.
Table 3 presents the results of the factor loadings on the TAM statements in Table 1. The factor loading is the correlation between a variable and a factor and the key to understanding the nature of a particular factor. In keeping with the TAM hypothesis, two clear factors emerged. The factor loadings were sufficiently high to cluster these variables into two distinct factors. As predicted by TAM, the first four variables were associated with the PU of a technology load together, and therefore, this factor is identified as PU. Also as predicted by TAM, factor loadings for easy to use and easy to understand load together. This factor is identified as PEOU.
Item loadings on the PU and PEOU factors for a nutrient management plan (NMP)
Variable | NMP | |||
---|---|---|---|---|
Factor 1 | Factor 2 | |||
| ||||
PU | Common variance | PEOU | Common variance | |
A nutrient management plan is … | ||||
Important to my farming needs | 0.7013 | 0.5 | 0.2709 | 0.07 |
Increases production | 0.8215 | 0.67 | 0.2304 | 0.05 |
Increases profits | 0.8368 | 0.7 | 0.1664 | 0.03 |
Is better than none | 0.7606 | 0.57 | 0.618 | 0.38 |
Saves time | 0.288 | 0.08 | 0.6353 | 0.4 |
Results are easy to understand | 0.1217 | 0.01 | 0.8909 | 0.79 |
Results are easy to use | 0.2741 | 0.07 | 0.7739 | 0.59 |
Percentage total variance | 0.37 | 0.33 | ||
Correlations between PU and PEOU | 0.59 | |||
KMO | 0.89 | |||
Cronbach’s alpha | 0.93 | 0.95 |
Values in bold represent the highest loading value for each statement on factor 1 and factor 2.
KMO = Kaiser–Meyer–Olkin; PU = perceived usefulness; PEOU = perceived ease of use.
The common variance is the squared factor loadings which indicate what percentage of the variance in the original variable is explained by a factor. For example, 50% of the variance in the statement “A nutrient management plan is important to my farming needs” is captured by factor 1 (PU), whereas factor 2 (PEOU) captures only 7% of the variance. On average, PU explains 37% of the variance in the dependent variable and PEOU explains 33%, PU and PEOU combined explain 70% of the total variance of the factor solution.
Table 3 also shows the Kaiser–Meyer–Olkin (KMO) measure of sampling adequacy. The KMO is an index that takes values between 0 and 1 and yields an assessment of whether variables belong together and have enough commonality to warrant a factor analysis (Dziuban & Shirkey, 1974). A KMO measure of factor suitability, an overall KMO of 0.89, indicates that the variables have sufficient commonality, which confirms factor analysis is an appropriate methodology. Consistent with other TAM literature, PU and PEOU are correlated with an average correlation of 59%. Cronbach’s alpha was also applied to assess the internal consistency or reliability of the composite scores (Cronbach, 1951; Cortina, 1993). When statements are used to form a composite score, the statements should all measure the same thing, and so they should be correlated with one another (Bland & Altman, 1997). Values for Cronbach’s alpha range from 0 to 1 and a value of 0.7 is considered adequate. Cronbach’s alpha for PU (0.933) and PEOU (0.951) indicates excellent internal reliability of the statements.
Ordered logit regression analysis
Having identified the two (factors) variables PU and PEOU were then used as the independent variables, in an ordered logit regression, where the dependent variable is the farmer’s statement “I intend to use a NMP for decision making in the next 12 months”. The results are presented in Table 4.
Ordered logit regression on intention to use a nutrient management plan (NMP) for decision-making in the next 12 mo
Intend to use NMP (dependent variable) | Coef | s.e. | Z | P > |z| |
---|---|---|---|---|
PU | 2.070*** | 0.187 | 11.077 | 0.000 |
PEOU | 1.636*** | 0.183 | 8.926 | 0.000 |
Cut 1 | −7.476*** | 0.557 | −13.425 | 0.000 |
Cut 2 | −5.400*** | 0.392 | −13.79 | 0.000 |
Cut 3 | −2.566*** | 0.227 | −11.305 | 0.000 |
Cut 4 | 1.381*** | 0.179 | 7.696 | 0.000 |
N | 358 | |||
Pseudo R-sq | 0.473 | |||
Standard errors in parentheses |
*** = P < 0.01.
PU = perceived usefulness; PEOU = perceived ease of use.
The results show that PU and PEOU are highly significant predictors of a farmer’s intention to use a NMP, but PU has a stronger effect. The Coef is the ordered log-odds estimate for a one unit increase in the independent variable on the expected level of the dependent variable, while other variables in the model are held constant. Therefore, if a farmer’s perception of the usefulness of a NMP were to increase by one category (strongly disagree to disagree), the ordered log-odds of their intention to adopt a NMP in the future would increase by 2.070; it would move up two categories. This is a significant finding and indicates the importance of the perception of the usefulness of a technology in a farmer’s future intention to adopt.
Results for PEOU are also highly significant, whereby a one unit increase in the PEOU of a NMP will result in a 1.636 unit increase in the ordered log-odds of being in a higher intention to use category.
In this study, there are five j alternatives: strongly agree, agree, neutral, disagree, strongly disagree ordered 1–5, and subsequently there are four cut-off or threshold points: cut 1–cut 4. Cut 1 is the threshold point between strongly agree and agree and so on. The cuts are the coefficients of the model in that response category.
Discussion
The adoption and use of a NMP are considered essential to managing farm practices that could reduce the risk of diffuse pollution from agriculture, while at the same time, saving on costs and improving profitability. However, farmer uptake of NMPs based on soil test results is less than one would expect, given the potential benefits for both farm profitability and water quality. The aim of this research was to investigate if a TAM could capture the strength and significance of farmer perceptions of a NMP prior to adopting the technology. The TAM maintains that the perception of a technology is highly influential and affects the attitude towards the technology and consequentially the future intention to adopt.
The strongest factor influencing future intention to adopt a NMP was the farmer’s PU of the NMP to his overall farming business. This variable captures a number of latent factors in relation to the usefulness including the economic motivations of the farmer. This highlights the importance of perceived economic benefit(s) as a driver of technology adoption. This finding is also consistent with previous TAM studies that found PU to be more significant that PEOU in terms of adoption and use (Flett et al., 2004; Kelly et al., 2016; McDonald et al., 2016). This result would indicate that in order to create a positive attitude towards the adoption of a NMP, policy makers and knowledge transfer specialists need to emphasise the usefulness of the technology both as a cost benefit and a time/labour saving tool to be implemented at the farm level.
While the PU of a NMP was the most important factor, PEOU which reflects farmer perceptions in relation to their own ability to adopt the technologies is also significant. Within the TAM it is hypothesised that PU is influenced by PEOU but not the other way round. Farmer perception in relation to the ease of use can positively influence the PU of a technology, but the two constructs are distinct from each other in that PU reflects the external effects of using a NMP on the day-to-day business of the farm, and PEOU reflects the internal belief that a farmer has in his own ability to use a NMP. The positive relationship between PEOU and a positive attitude towards adoption emphasises the need for clear guidelines and information to be provided to farmers on how to best use a NMP.
The research recommends that building on the results of the TAM the usefulness of a NMP, in terms of increased profitability, improving nutrient management practices, labour and time-saving advantages should be highlighted and clearly communicated to farmers. This will help to influence their PU of the technology in a positive way and hence improve the probability of future adoption. Hand in hand with the information to enhance a farmer’s PU information farmers should also be given practical information on how to use the NMP for their farm. This could include one-to-one instruction from the farmers’ knowledge transfer specialist, in-field demonstrations and free trials coupled with support services such as videos to show how to use the plan to achieve the best results for the farm. This aspect builds on the importance of a farmer’s PEOU of the technology in affecting their future intention to adopt, as highlighted in the TAM.